Medical Image Annotation: The Key to Advancing Healthcare Technology
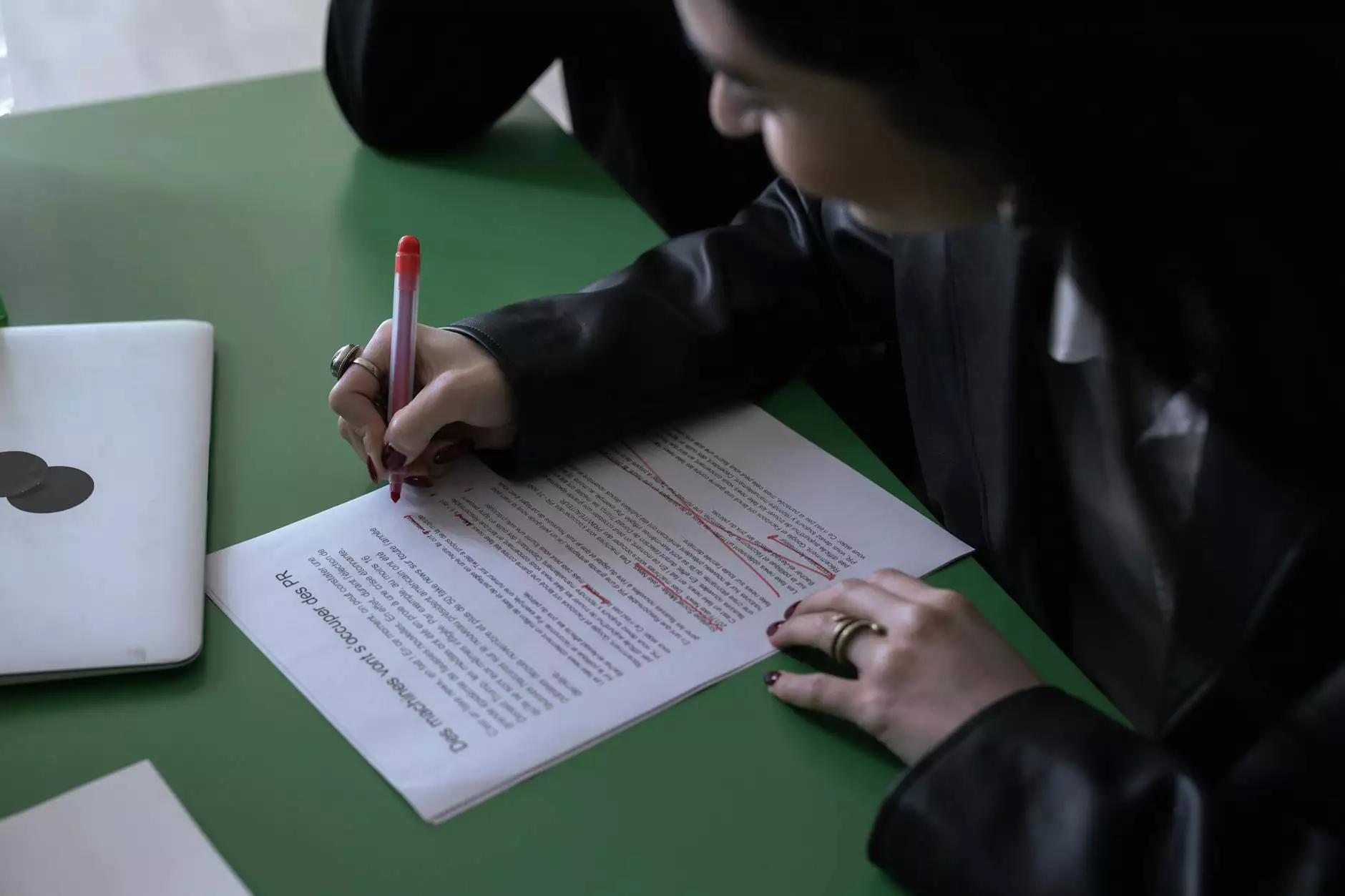
In the ever-evolving field of healthcare technology, the significance of medical image annotation cannot be overstated. By transforming complex medical images into actionable data, this essential process is paving the way for enhanced diagnostic accuracy and improved patient outcomes. In this comprehensive article, we will delve deeply into the intricacies of medical image annotation, its processes, various applications, and the undeniable benefits it brings to the medical community.
Understanding Medical Image Annotation
At its core, medical image annotation refers to the process of identifying and labeling various components within medical images, such as X-rays, MRIs, and CT scans. This process can involve delineating structures within images or providing contextual information about pathologies, anatomical landmarks, and other significant features. The goal is to create a set of metadata that can be used for further analysis, training AI algorithms, and supporting clinical decisions.
The Importance of Medical Image Annotation in AI and Machine Learning
Artificial Intelligence (AI) and Machine Learning (ML) are transforming multiple sectors, and healthcare is no exception. Annotated medical images serve as the backbone for training AI systems. Here’s why:
- Improved Diagnostic Tools: AI algorithms rely on high-quality, annotated datasets to accurately identify conditions from images.
- Efficiency in Healthcare Delivery: Automated annotation can streamline workflows, reducing the time clinicians spend diagnosing patients.
- Continuous Learning: Ongoing data collection and annotation can help refine AI models, adapting to new challenges and improvements in medical understanding.
The Process of Medical Image Annotation
The medical image annotation process can be complex and multifaceted. Below are the key steps involved:
- Image Acquisition: Obtaining high-quality medical images through various imaging modalities like MRI, CT, or ultrasound.
- Selection of Annotation Tools: Choosing appropriate software tools designed for medical image annotation, capable of various functionalities to suit specific requirements.
- Defining Annotation Guidelines: Establishing clear protocols that dictate how annotations should be performed to ensure consistency and accuracy.
- Annotation Process: Trained professionals, often radiologists or trained annotators, meticulously label images, marking areas of interest.
- Quality Control: Implementing a robust review system to ensure annotations meet predefined standards and are free from errors.
- Data Integration: Integrating annotated data into a centralized database to be accessible for AI training and research.
Types of Medical Image Annotations
Medical image annotations can take various forms based on the objective of the study or treatment:
- Bounding Boxes: These are rectangular, labeled regions that highlight critical areas in an image, often used in localization problems.
- Segmentation: Involves partitioning an image into regions or segments to pinpoint precise anatomical structures.
- Point Annotations: Specific coordinates marked on images to indicate locations of interest, such as tumors.
- 3D Annotations: In multidimensional imaging, it involves creating volumetric datasets that can provide more comprehensive insights.
Applications of Medical Image Annotation
The applications of medical image annotation stretch across various domains within healthcare. Here are some noteworthy examples:
Radiology
In radiology, annotated images assist in diagnosing conditions such as cancer by identifying signs that may be overlooked. This leads to timely intervention and better patient prognosis.
Pathology
In pathology, image annotation is crucial for identifying cellular structures in microscopic images, aiding in the diagnosis of diseases like cancer.
Surgery
Annotated images are instrumental in planning surgical procedures, providing surgeons with insights into the anatomy they will encounter.
Research and Development
Clinics and research institutions utilize annotated datasets to develop new diagnostic tools and improve existing technologies, thus driving the evolution of healthcare methods.
The Benefits of Medical Image Annotation
The advantages of implementing effective medical image annotation processes are vast:
- Enhanced Accuracy: With precise annotations, healthcare professionals can rely on accurate data to make informed decisions, leading to better outcomes.
- Speedy Diagnosis: Quick access to annotated images can considerably decrease the time taken to arrive at a diagnosis.
- Better Training for AI Models: Well-annotated datasets enhance the quality of AI training, resulting in robust tools capable of making reliable predictions.
- Reduced Human Error: Annotations that are standardized and validated help minimize discrepancies in clinical diagnoses.
Challenges in Medical Image Annotation
Despite the many advantages, there are challenges associated with medical image annotation:
- Labor-Intensive Process: The need for skilled annotators can lead to increased costs and extended timelines.
- Inter-Annotator Variability: Different annotators might not always provide consistent annotations, leading to variability in data.
- Data Privacy Concerns: Handling sensitive medical data requires strict compliance with regulations, complicating the annotation process.
The Future of Medical Image Annotation
The future of medical image annotation is promising, with several trends shaping its trajectory:
- Increased AI Integration: Enhanced models capable of semi-automated annotation processes will reduce manual intervention and increase efficiency.
- Standardization of Practices: Establishing industry standards for annotation will ensure consistency and reliability across institutions.
- Real-Time Annotation: The development of technologies enabling real-time annotations during procedures will revolutionize surgical practices.
- Cross-Disciplinary Collaboration: Collaborations between software developers, healthcare professionals, and researchers will enhance the quality and efficiency of annotation processes.
Conclusion
In summary, medical image annotation plays a pivotal role in contemporary healthcare, driving improvements in diagnostic accuracy and efficiency. Its impact is felt across various medical fields, empowering clinicians and researchers alike. With ongoing advancements in technology and AI integration, the future holds tremendous promise for the evolution and implementation of medical image annotation, ultimately leading to better patient care and outcomes. As stakeholders in the healthcare sector, we should embrace these innovations and strive for continuous improvement in diagnostic practices.
For cutting-edge software development in the field of medical image annotation, businesses like Keymakr are leading the charge, combining expertise with technology to revolutionize how medical images are annotated, thus enhancing the overall healthcare delivery process.